机器学习实操 第一部分 机器学习基础 第4章 训练模型
内容概要
第4章深入探讨了训练模型的关键技术和算法。从线性回归模型的经典训练方法(如正规方程和梯度下降)到多项式回归和正则化技术,本章提供了丰富的理论和实践知识。此外,还介绍了逻辑回归和Softmax回归,用于解决分类问题。通过这些内容,读者将掌握如何选择合适的模型、训练算法和超参数,以应对各种机器学习任务。
主要内容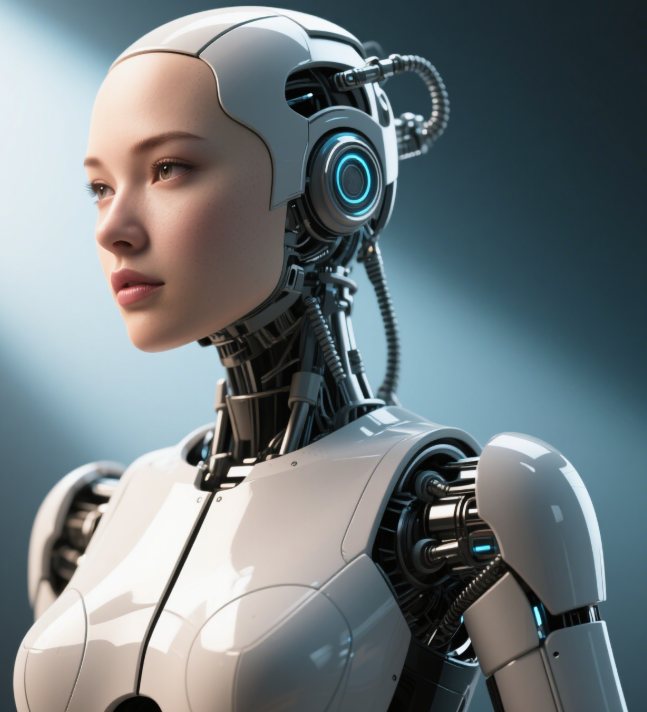
-
线性回归
- 正规方程:通过闭式解直接计算最优模型参数。
- 梯度下降:通过迭代优化方法逐步调整模型参数以最小化成本函数,包括批量梯度下降、随机梯度下降和小批量梯度下降。
-
多项式回归
- 特征变换:通过添加多项式特征将线性模型扩展到非线性数据。
- 学习曲线:通过绘制训练误差和验证误差随训练迭代次数的变化曲线,判断模型是否过拟合或欠拟合。
-
正则化技术
- 岭回归:通过添加L2正则化项约束模型权重,减少过拟合。
- Lasso回归:通过添加L1正则化项进行特征选择,输出稀疏模型。
- 弹性网络回归:结合L1和L2正则化,平衡特征选择和权重约束。
- 提前停止:在梯度下降过程中,当验证误差达到最小值时停止训练,防止过拟合。
-
逻辑回归
- 概率估计:通过逻辑函数(sigmoid函数)将线性回归模型的输出转换为概率。
- 决策边界:通过设置概率阈值进行分类决策。
-
Softmax回归
- 多类分类:将逻辑回归扩展到多类分类问题,通过Softmax函数计算每个类别的概率。
- 交叉熵损失:用于衡量模型预测概率与真实标签的差异,指导模型训练。
关键代码和算法
4.1 线性回归与正规方程
import numpy as np
from sklearn.linear_model import LinearRegression# 生成线性数据
np.random.seed(42)
m = 100
X = 2 * np.random.rand(m, 1)
y = 4 + 3 * X + np.random.randn(m, 1)# 使用正规方程计算最优参数
from sklearn.preprocessing import add_dummy_feature
X_b = add_dummy_feature(X)
theta_best = np.linalg.inv(X_b.T @ X_b) @ X_b.T @ y# 使用Scikit-Learn进行线性回归
lin_reg = LinearRegression()
lin_reg.fit(X, y)
4.2 梯度下降
# 批量梯度下降
eta = 0.1
n_epochs = 1000
m = len(X_b)
np.random.seed(42)
theta = np.random.randn(2, 1)for epoch in range(n_epochs):gradients = 2 / m * X_b.T @ (X_b @ theta - y)theta = theta - eta * gradients# 随机梯度下降
n_epochs = 50
t0, t1 = 5, 50def learning_schedule(t):return t0 / (t + t1)np.random.seed(42)
theta = np.random.randn(2, 1)for epoch in range(n_epochs):for iteration in range(m):random_index = np.random.randint(m)xi = X_b[random_index:random_index+1]yi = y[random_index:random_index+1]gradients = 2 * xi.T @ (xi @ theta - yi)eta = learning_schedule(epoch * m + iteration)theta = theta - eta * gradients
4.3 多项式回归
from sklearn.preprocessing import PolynomialFeatures# 生成非线性数据
np.random.seed(42)
m = 100
X = 6 * np.random.rand(m, 1) - 3
y = 0.5 * X**2 + X + 2 + np.random.randn(m, 1)# 添加多项式特征
poly_features = PolynomialFeatures(degree=2, include_bias=False)
X_poly = poly_features.fit_transform(X)# 线性回归拟合多项式特征
lin_reg = LinearRegression()
lin_reg.fit(X_poly, y)
4.4 正则化技术
from sklearn.linear_model import Ridge, Lasso, ElasticNet# 岭回归
ridge_reg = Ridge(alpha=0.1, solver="cholesky")
ridge_reg.fit(X, y)# Lasso回归
lasso_reg = Lasso(alpha=0.1)
lasso_reg.fit(X, y)# 弹性网络回归
elastic_net = ElasticNet(alpha=0.1, l1_ratio=0.5)
elastic_net.fit(X, y)
4.5 逻辑回归与Softmax回归
from sklearn.linear_model import LogisticRegression# 逻辑回归
log_reg = LogisticRegression(random_state=42)
log_reg.fit(X_train, y_train)# Softmax回归
softmax_reg = LogisticRegression(C=30, random_state=42)
softmax_reg.fit(X_train, y_train)
精彩语录
-
中文:理解模型的工作原理可以帮助你快速确定合适的模型、训练算法和超参数。
英文原文:Having a good understanding of how things work can help you quickly home in on the appropriate model, the right training algorithm to use, and a good set of hyperparameters for your task.
解释:强调了理解模型内部机制的重要性。 -
中文:梯度下降是一种通用优化算法,能够找到多种问题的最优解。
英文原文:Gradient descent is a generic optimization algorithm capable of finding optimal solutions to a wide range of problems.
解释:介绍了梯度下降的广泛应用。 -
中文:在处理大量特征或训练实例时,梯度下降比正规方程更高效。
英文原文:Gradient descent scales well with the number of features; training a linear regression model when there are hundreds of thousands of features is much faster using gradient descent than using the Normal equation or SVD decomposition.
解释:对比了梯度下降和正规方程的计算复杂度。 -
中文:正则化是减少过拟合的有效方法,通过约束模型权重来实现。
英文原文:A good way to reduce overfitting is to regularize the model (i.e., to constrain it): the fewer degrees of freedom it has, the harder it will be for it to overfit the data.
解释:说明了正则化的作用。 -
中文:逻辑回归和Softmax回归是解决分类问题的有力工具,能够输出概率估计。
英文原文:Logistic regression and softmax regression are powerful tools for classification tasks, providing estimated probabilities for each class.
解释:强调了逻辑回归和Softmax回归在分类任务中的应用。
###总结
通过本章的学习,读者将掌握线性模型的训练方法,包括线性回归、多项式回归、正则化技术和逻辑回归。这些技术涵盖了从简单的线性关系到复杂的非线性关系的建模,以及从二元分类到多类分类的处理。理解这些方法的原理和实现,将为读者在实际项目中应用和优化机器学习模型打下坚实基础。