1.CLIP (Contrastive Language–Image Pre-training)
笔记来源:
1.How does Stable Diffusion work?
2.CLIP: Connecting text and images
1.1 Introduction
CLIP 在 Stable Diffusion 中的位置(右侧橙色框)
CLIP将text转化为embedding的目的是用文本引导噪声预测器unet,以便预测的噪声在从图像中减去后能够为我们提供我们想要的结果。
The output of the text transformer is used multiple times by the noise predictor throughout the U-Net.
text到embedding的流程
(1) Tokenizer first converts each word in the prompt to a number called a token.
(2) Each token is then converted to a 768-value vector called embedding.
(3) The embeddings are then processed by the text transformer and are ready to be consumed by the noise predictor.
CLIP将text转化为embedding后与image latent 和 timestep embedding 共同注入到unet中
1.2 Tokenizer
使用Embedding之前,我们可以直接调用transformer的CLIPtokenizer,先将text转化为token,例如:
from transformers import CLIPTokenizer
# Input text
text = "A panda is eating bamboo."
tokenizer = CLIPTokenizer("/home/wxy/Documents/PycharmProjects/pytorch-stable-diffusion/sd/data/tokenizer_vocab.json","/home/wxy/Documents/PycharmProjects/pytorch-stable-diffusion/sd/data/tokenizer_merges.txt")
# Convert into af list of length seq_len=77
# Tokenize the text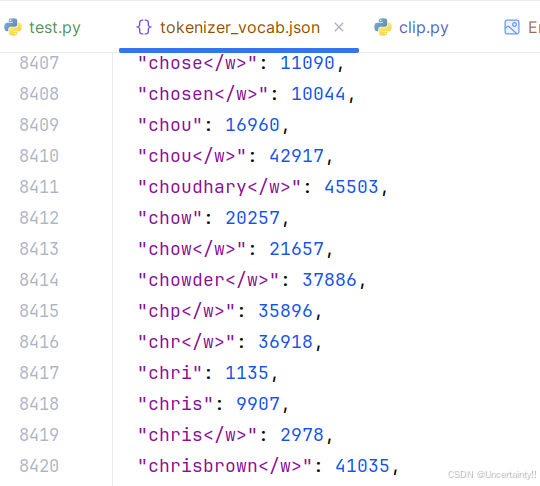tokens = tokenizer.encode_plus(text,add_special_tokens=True, # Adds [CLS] and [SEP] tokensmax_length=77, # Maximum length of the sequencepadding='max_length', # Pads to the maximum lengthtruncation=True, # Truncates if the text is longer than max_lengthreturn_tensors='pt' # Returns PyTorch tensors
).input_ids.to(device)
tokens_tensors = tokens.to(torch.long)
tokenizer_vocab.json
The primary role of “tokenizer_vocab.json” is to define the vocabulary used by the tokenizer. The vocabulary consists of a mapping between tokens (which can be words, subwords, or characters) and their corresponding unique integer IDs.
tokenizer_merges.text
The primary role of “tokenizer_merges.txt” is to define the sequence of merge operations used to build the subword vocabulary from individual characters or smaller subunits.
1.3 CLIP Embedding
class CLIPEmbedding(nn.Module):def __init__(self, n_vocab: int, n_embd: int, n_token: int):super().__init__()# Token embedding layerself.token_embedding = nn.Embedding(n_vocab, n_embd)# Position embedding parameter (learnable)# A learnable weight matrix encodes the position information for each tokenself.position_embedding = nn.Parameter(torch.zeros((n_token, n_embd)))# This initializes a parameter tensor with zeros of shape (n_token, n_embd).# nn.Parameter makes it trainable by PyTorch.def forward(self, tokens):# (Batch_Size, Seq_Len) -> (Batch_Size, Seq_Len, Dim)# Token embeddings lookupx = self.token_embedding(tokens)# Add position embeddings# (Batch_Size, Seq_Len) -> (Batch_Size, Seq_Len, Dim)x += self.position_embedding # position for each token# self.position_embedding will be added to each element in x.return x
1.4 CLIP Layer (LN+SelfAttention)
class CLIPLayer(nn.Module):def __init__(self, n_head: int, n_embd: int):super().__init__()# Pre-attention normself.layernorm_1 = nn.LayerNorm(n_embd)# Self attentionself.attention = SelfAttention(n_head, n_embd)# Pre-FNN normself.layernorm_2 = nn.LayerNorm(n_embd)# Feedforward layerself.linear_1 = nn.Linear(n_embd, 4 * n_embd)self.linear_2 = nn.Linear(4 * n_embd, n_embd)def forward(self, x):# (Batch_Size, Seq_Len, Dim)residue = x### SELF ATTENTION #### (Batch_Size, Seq_Len, Dim) -> (Batch_Size, Seq_Len, Dim)x = self.layernorm_1(x)# (Batch_Size, Seq_Len, Dim) -> (Batch_Size, Seq_Len, Dim)x = self.attention(x, causal_mask=True)# (Batch_Size, Seq_Len, Dim) + (Batch_Size, Seq_Len, Dim) -> (Batch_Size, Seq_Len, Dim)x += residue### FEEDFORWARD LAYER #### Apply a feedforward layer where the hidden dimension is 4 times the embedding dimension. residue = x# (Batch_Size, Seq_Len, Dim) -> (Batch_Size, Seq_Len, Dim)x = self.layernorm_2(x)# (Batch_Size, Seq_Len, Dim) -> (Batch_Size, Seq_Len, 4 * Dim)x = self.linear_1(x)# (Batch_Size, Seq_Len, 4 * Dim) -> (Batch_Size, Seq_Len, 4 * Dim)x = x * torch.sigmoid(1.702 * x) # QuickGELU activation function# (Batch_Size, Seq_Len, 4 * Dim) -> (Batch_Size, Seq_Len, Dim)x = self.linear_2(x)# (Batch_Size, Seq_Len, Dim) + (Batch_Size, Seq_Len, Dim) -> (Batch_Size, Seq_Len, Dim)x += residuereturn x
1.5 CLIP
class CLIP(nn.Module):def __init__(self):super().__init__()# CLIPEmbedding layer to convert token indices into embeddingsself.embedding = CLIPEmbedding(49408, 768, 77)# List of CLIPLayer modules for multi-layer processingself.layers = nn.ModuleList([CLIPLayer(12, 768) for i in range(12)])# Layer normalization layerself.layernorm = nn.LayerNorm(768)def forward(self, tokens: torch.LongTensor) -> torch.FloatTensor:# Ensure tokens are of type longtokens = tokens.type(torch.long)# Embedding layer: (Batch_Size, Seq_Len) -> (Batch_Size, Seq_Len, Dim)# (Batch_Size, Seq_Len) -> (Batch_Size, Seq_Len, Dim)state = self.embedding(tokens)# Apply multiple CLIPLayer modules sequentially# Apply encoder layers similar to the Transformer's encoder.for layer in self.layers:# Layer application# (Batch_Size, Seq_Len, Dim) -> (Batch_Size, Seq_Len, Dim)state = layer(state)# Apply layer normalization# (Batch_Size, Seq_Len, Dim) -> (Batch_Size, Seq_Len, Dim)output = self.layernorm(state)return output